Data governance policy, data risk management framework, data risk management handbook, data risk training, data quality control standards.
Read more about benefits.
Data Governance, Quality and Risk
Data Quality Works is a consulting firm specialising in data governance, quality, and risk. We create and implement data governance, quality, and risk policies and frameworks, evaluate and roll out data governance tools, build business glossaries, and ensure high data quality during migrations and data platform development.
Reach out at info@dataqualityworks.com or book an introductory call to discuss.
Services
Critical Data Element register, business glossary, data lineage, data quality dashboards and control assessment documentation.
Read more about benefits.
Data governance tool requirements, recommendation papers, pilot plans, solution design, implementation.
Read more about benefits.
Custom metadata model requirements, solution design, custom metadata scanner source code integrating with a data governance tool, data governance tool configuration.
Read more about benefits.
Data migration risk assessments, test plans, machine-readable source to target mapping, automated testing framework, test reporting.
Read more about benefits.
Posts, Presentations and Open Source Projects
Prototyping a data quality analytics solution with Microsoft Fabric and Power BI highlighted a couple of possible approaches to bridge the gaps in the existing data quality tools - a dimensional model for data quality measures and a compact data lineage view.
Yoke Yee Loo, Roberto Sammassimo, and I recently discussed making data governance conversations tangible. We all agreed that the language of risk resonates well, but what is the next level of detail required to translate it into action? This short article has three ideas to help with the implementation.
There are many ways to organise resources in Microsoft Purview Classic, from Glossaries to Classifications, Tags, Sensitivity Labels, Collections, and more. This post provides an at-a-glance view of their interaction.
Building Business Glossaries in Microsoft Purview without clear requirements and without making upfront design decisions will cause pain later on. The critical decisions are whether to catalogue concepts or terms, how to deal with facets, and how to structure glossaries based on who has the authority over definitions.
Developing a business glossary using faceted classification, a set of hierarchies across mutually exclusive fundamental concepts, can effectively connect business language with dimensional data quality measurements.
Taxonomies for Confluence add-on is now available under the MIT license from Github.
Somewhat contrarian data beliefs and their consequences for implementing data governance. These beliefs are about definitions of data and information, aboutness, classification and metaphors.
Outline of the foldable 3D shape to help communicate faceted classification ideas.
SKOS version of Australian and New Zealand Standard Industrial Classification (ANZSIC) that can be loaded into SKOS-compatible taxonomy tools.
Capturing capability models using TOGAF Metamodel Ontology provides an excellent opportunity to visualise, share and debate them.
The purpose of this demo presented at the Data Centric Architecture Forum 2020 is to show capturing of the provenance information using common vocabulary of PROV-O in a repo trading and risk reporting scenario.
Looking into benefits and challenges of developing harmonised regulatory taxonomies, benefits in the absence of such harmonisation, and the role that regulators, regulated firms and vendors can play in the taxonomy development.
Looking into the main beneficiaries of Model Driven Machine Executable Regulation (MDMER), and the role of third party service providers in its adoption.
RdfPandas is a module providing RDF support for Pandas. It consists of two simple functions for Graph to DataFrame conversion and DataFrame to Graph conversion.
Building software performance testing analytics pipeline involves defining the analysis question, getting and cleaning data, performing analysis and integrating the results with the testing frameworks.
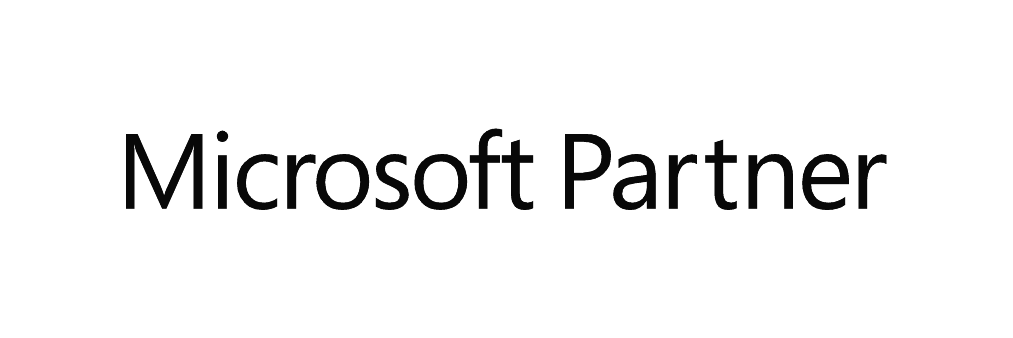